Data, data everywhere and not a byte to eat
Data, data everywhere and not a byte to eat
Identifying āthingsā
Simple question: what is this thing?
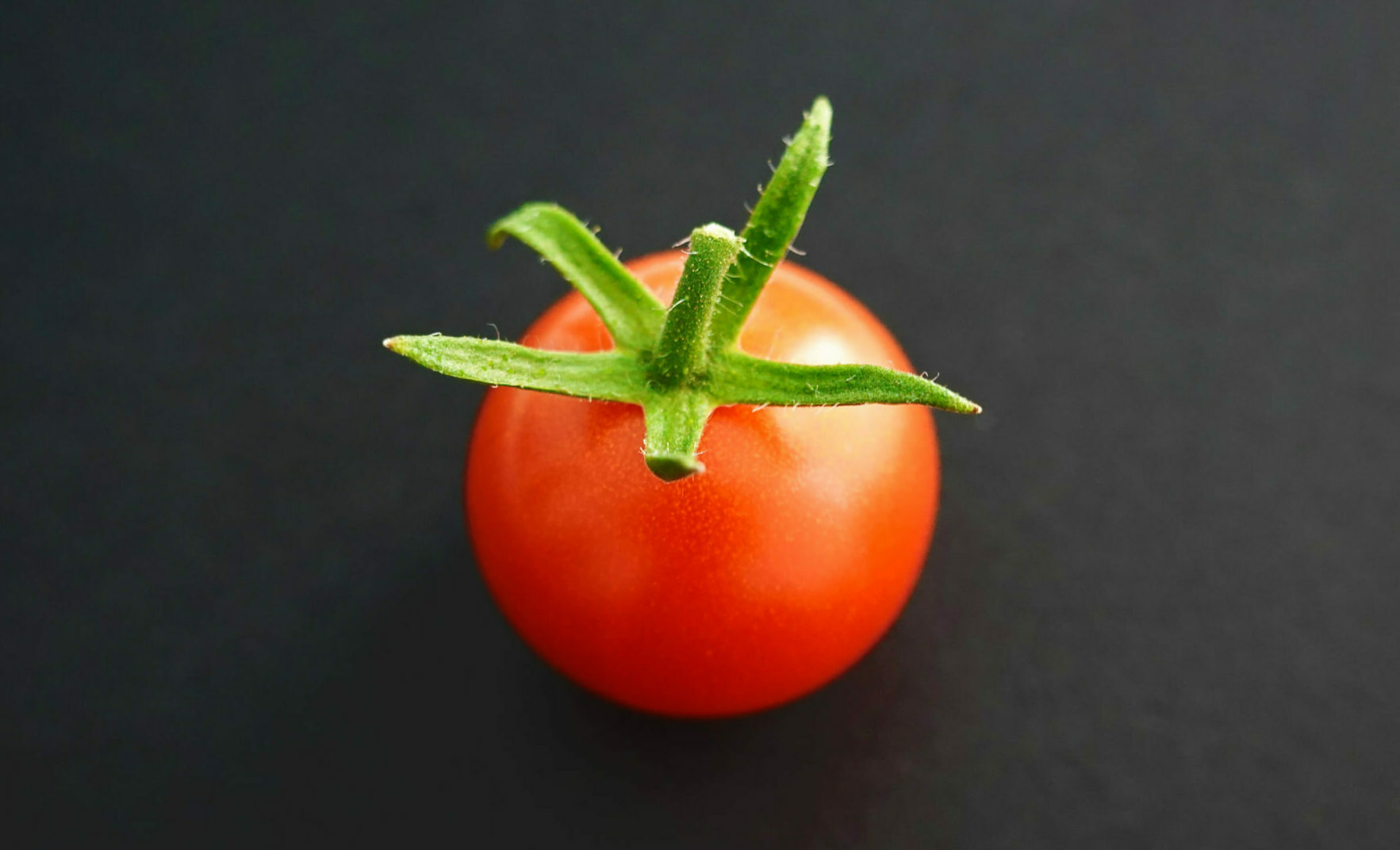
Itās a tomato, of course. Iām certain of it, so are you. But how do we know itās a tomato by just looking at it? What is it about this little red āthingā that lets us know that itās a tomato?
A tomato has attributes and visual characteristics that our eyes pass to the brain to process and categorise against a ādatabaseā of information that we previously learned. Like everything else that exists, the tomato has distinguishing attributes: itās red, round, bulbous, shiny, and it has a green stem protruding from an indent on top of it. All these data points come together to provide us with the necessary information to determine that it is most likely a tomato.
Ok, then, what is this thing?
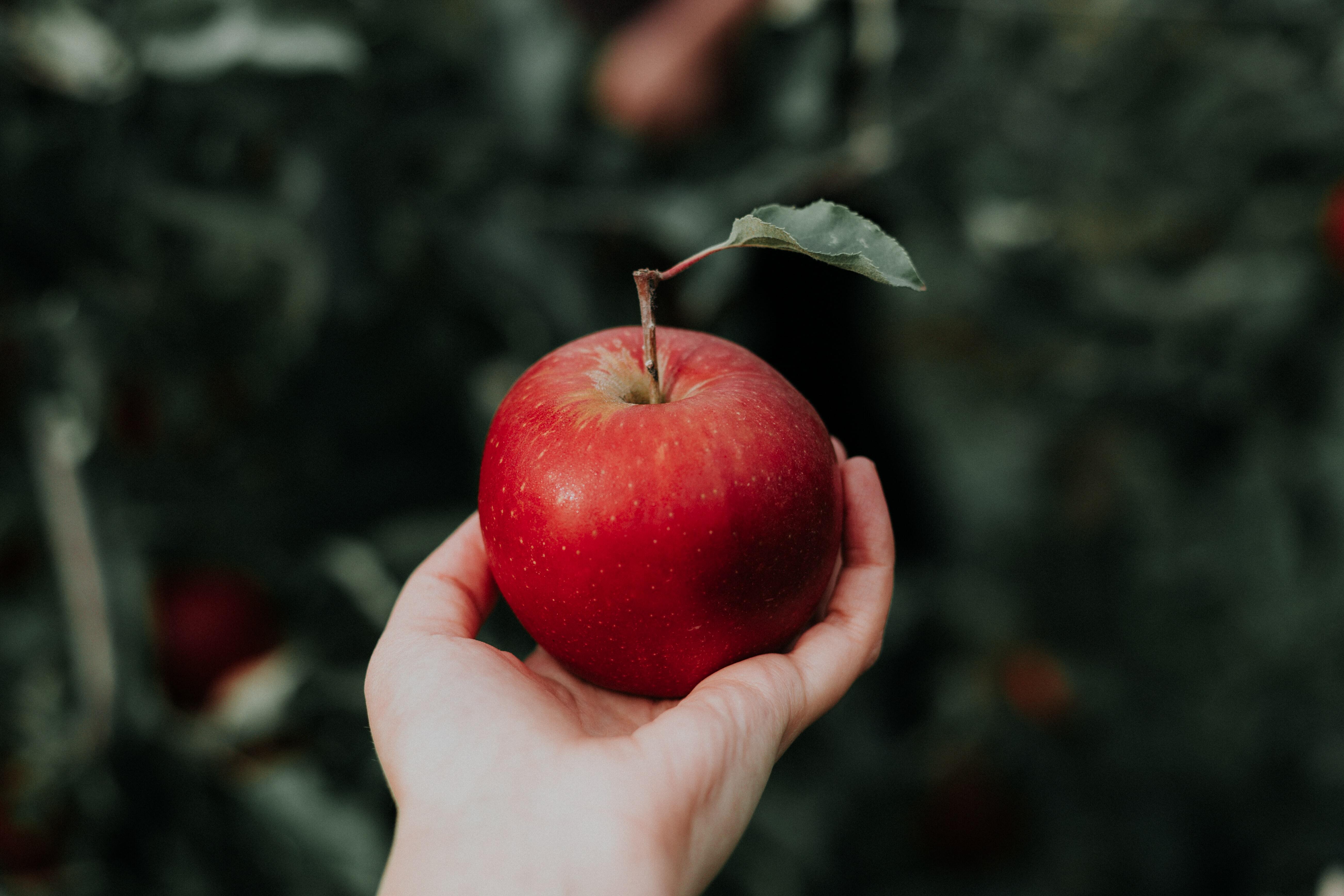
Itās also red, round, bulbous, shiny, and it has a green stem protruding from an indent on top of it. So is it another tomato? Hang on, its shape is a little different: the colour is red, but itās a different shade of red; the colour is not uniform, there are little pale dots; the shoot is different, and the skin texture is a little more glossy. Combined, all these nuances in data provide us with the information, which, in turn, enables us to determine that itās an apple.
So, what about these other round, red things?
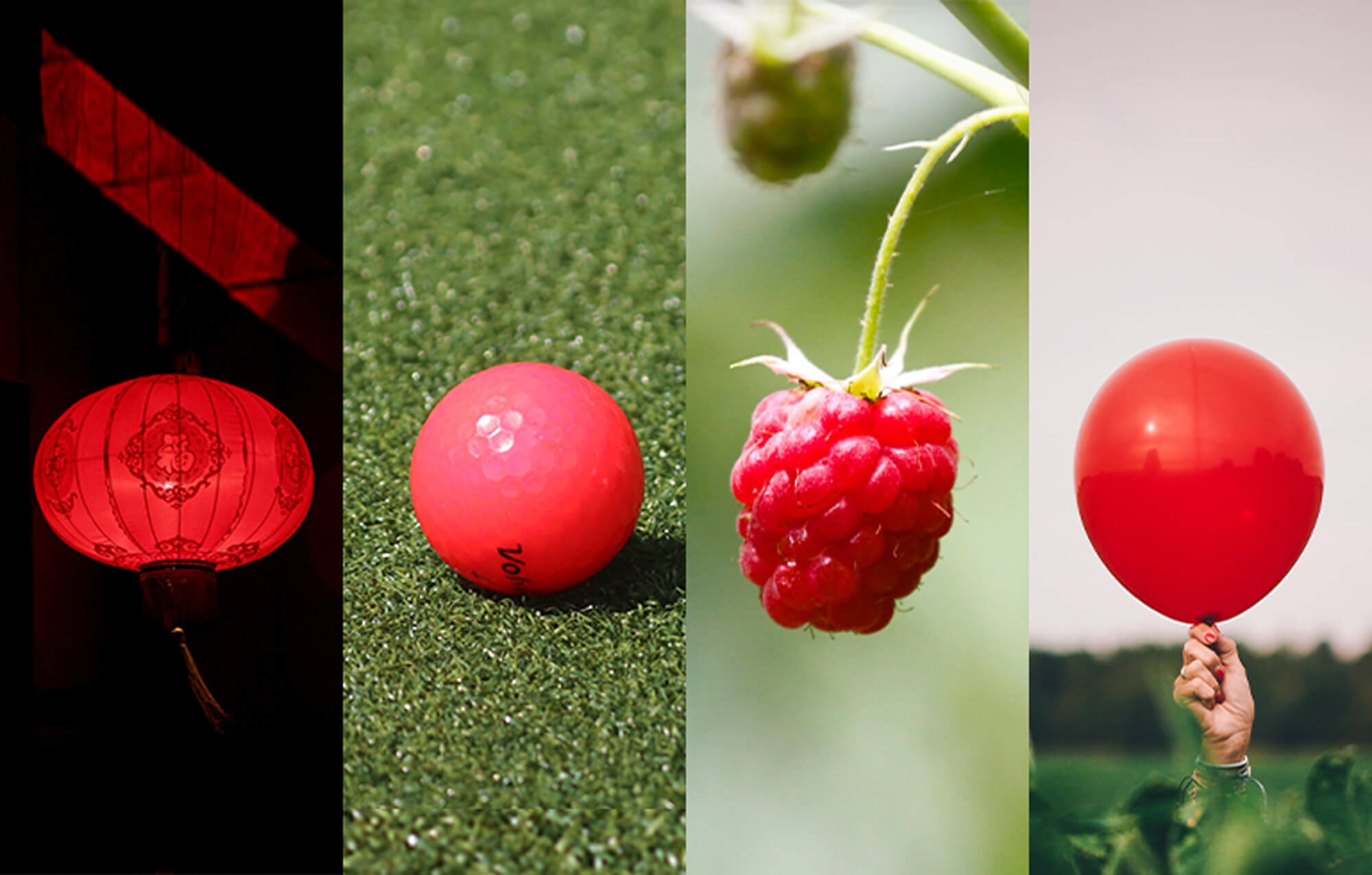
The brain can detect properties very quickly, which enables us to identify that these āthingsā are different to red tomatoes or red apples. For simple examples, such as those mentioned above, the brain computes this instantly because it classified these slight differences in object attributes as distinct āthingsā from a young age through trial and error and now they are familiar to us. We also learned the properties of an objectās state through our senses: Is it too hot to touch? Is it OK to eat or is it rotten? - my sight and smell detectors might say otherwise!
Why are we talking about tomatoes and apples and what does it have to do with the built environment and digital twins? Well, if we consider the elements that combine to make a building, they are no different: each has attributes that help us identify them.
Learned databases and building components
If we apply the same concept to the objects and equipment you typically find in commercial real estate, for those who work in the industry, the brain identifies items based on shape, size, colour, configuration, and perceived function. In the following images, the toilet and the door are familiar and easy to identify; however, our brain may struggle to categorise the data we receive about the remaining four items because the data we receive is not complete enough to tell us what they are when compared to our database. Simply put, we donāt recognise them because we have not learned what they are. If we donāt know what these items are, then we cannot use them as designed, we cannot interact with them, and we cannot share them effectively with others. We need more contextual data about them so we can identify them and we can make informed decisions.
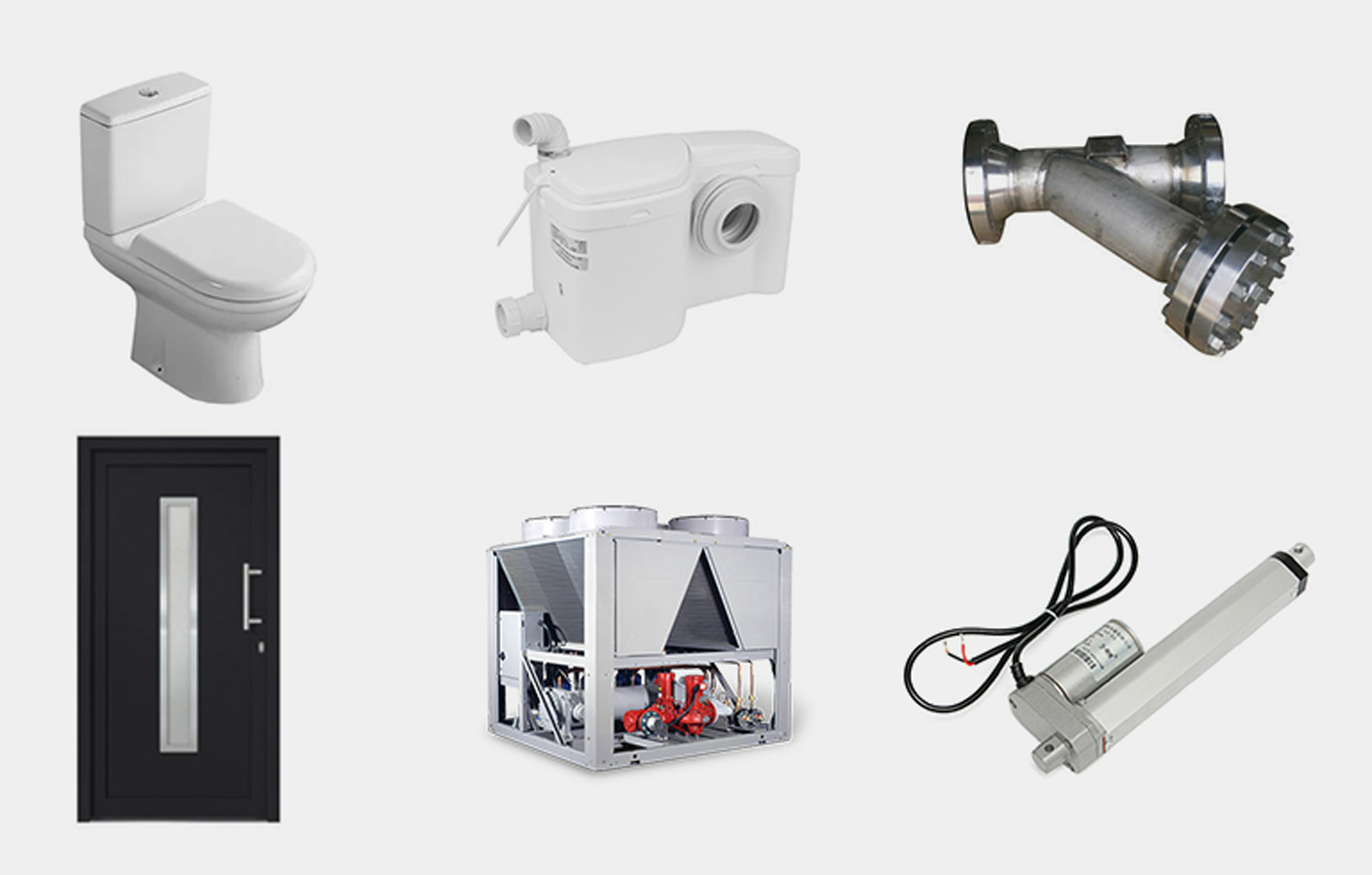
To dig a little deeper, the brain has a contextual database: if we see something, we automatically put that āthingā into context and automatically associate it to other āthingsā. Scientists at John Hopkins University and the University of Pennsylvania found that if a person sees an object, like a toothbrush, they subconsciously associate that object with a bathroom, a mirror, a sink, and so forth; in our analogy, you might subconsciously associate a tomato with tomato sauce, basil, spaghetti bolognese, and pizza. Returning to the built environment, along with identifying building items, we must also contextualise this data and relate it to other items.
The identifying data we want and why we contextualise it
Returning to the unidentified āthingsā above, if I said they were a āchillerā, āstrainerā, āmaceratorā and āactuatorā, would you be any wiser as to what these things are? Maybe thatās enough data for some people, but most need more data. We need the following context:
- What is their function?
- Where are these items located?
- Are they connected to or related to any other items?
- What system are they part of?
This data gives us context. Now we have more relevant data about these objects and our brain has a better understanding of what they are and how to interact with them: Is it OK to touch or is it dangerously hot? How long before this item expires and what parts of the system will its expiration affect?
Having the āwhatā and āwhereā data alongside the contextual data means that we can answer much more than āwhat is it?ā. For example, we can answer the following questions:
- What floor and room do I go to address a blocked toilet fault?
- I need to replace a door, does the new one need a specific fire rating?
- I need someone to service my chiller, who should I call? What make and model is it and where is the manual they can use to fix it?
- I need to get my strainers cleaned out, where are they?
- My kitchen macerator is broken, is the old one within warranty?
- Which of my air filters need replacing and when?
- When I run my fire alarm test, which vents are going to open automatically?
Machine readability: the computer as a brain
The computer is based on the brain but unlike our plastic brain, which learns through experience, the computer must be spoon-fed the data and told its context. Most companies use software to design, construct and operate their built assets; without data, these technologies wonāt work. The process of structuring data in a contextual manner enables software technologies to use and understand it. In essence, we humans create the learned database for the ādumbā technology so that it can do what it does best: compute and gain insights from large volumes of data. This makes us much more efficient at our jobs, and makes our buildings and their components operate automatically.
Accurate data
However, there is a caveat: the accuracy of the data is fundamental to its usage; or, to coin a phrase from the early days of computing: garbage in, garbage out. In 2011, a Gartner study found that 40% of the anticipated value of all business initiatives is never achieved and that poor data quality in both the planning and execution phases of these initiatives is a primary cause. This means that validating and verifying data is as important as getting data; having unchecked data means you donāt have data.
I hope you enjoyed reading this blog, which was a bit of fun. Why not think about what data you really have about a building and what data would be wise to have.
After all, āKnowledge is knowing that the tomato is a fruit, wisdom is knowing not to put it in a fruit salad.ā - Miles Kington
Iām a solution consultant at Invicara. Reach out to me if you would like to have a conversation. john.dillon@invicara.com